Data and healthcare are two terms that initially appear incompatible, as healthcare implies a large volume of sensitive data. However, Big Data in the healthcare field is on a roll.
According to the “Global Big Data in Healthcare Market: Analysis and Forecast, 2017–2025”, Big Data in the healthcare industry is expected to reach $68.75 billion in 2025.
Mining a tremendous potential
Big Data is a game changer in many areas such as industry, environmental management, transportation, and education. However, the most promising area where Big Data can make a significant difference is healthcare. Big Data analytics in healthcare presents an enormous potential in terms of avoiding preventable diseases, reducing the costs of treatment, predicting epidemic outbreaks, and overall improving the quality of life.
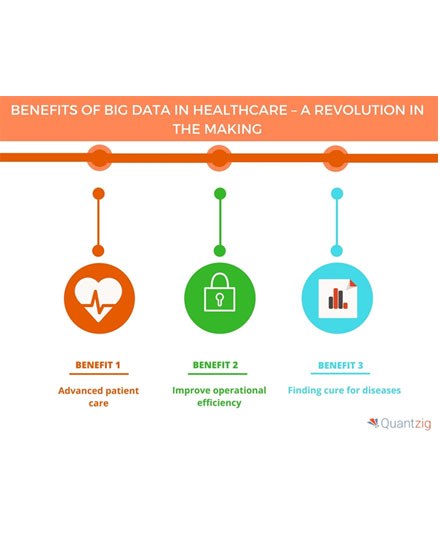
As a McKinsey report states that after over 20 years of steady increases, healthcare expenses currently represent “17.6% of GDP—nearly $600 billion more than the expected benchmark for a nation of the United States’ size and wealth.” In other words, the opportunities to maximize healthcare expenses are massive, and healthcare analytics are an effective means of reducing some expenses.
Developing prevention via health tracking
A major opportunity is the prevention of diseases through patient health tracking. Monitoring heart rate, sleep habits, blood pressure, and glucose levels is now possible for specialists. In previous years, doctors used to acquire such information during a consultation or a blood test. Today they can obtain data from day-to-day life, which is highly critical for preventing certain diseases.
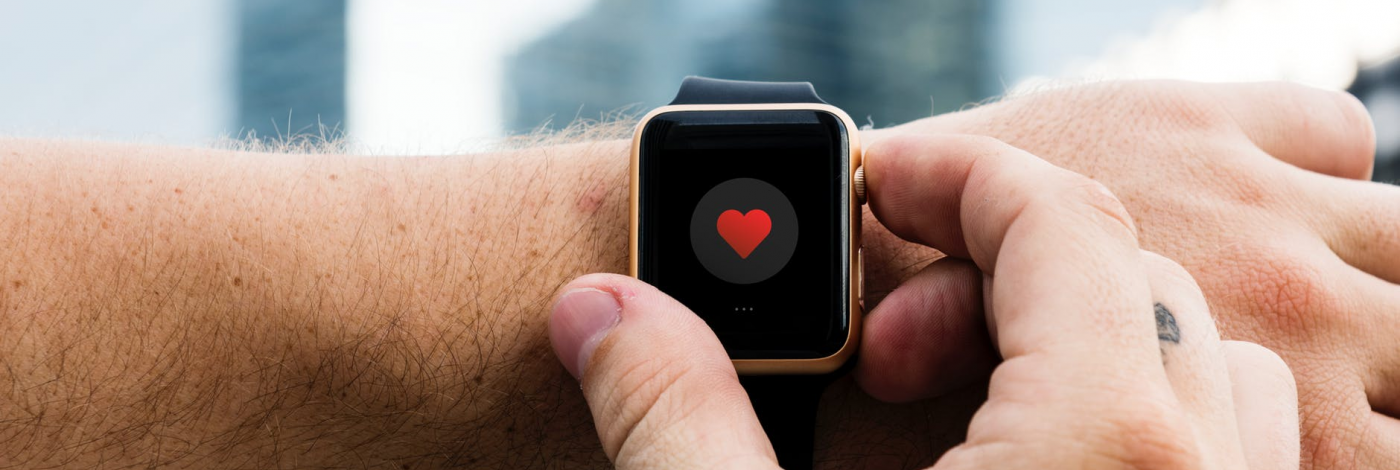
Apple’s smartwatch, iWatch, is capable of monitoring heart rate and detecting irregular heartbeats. Doctors can exploit such data.
Predictive healthcare
Another opportunity is predictive analysis, which allows for identifying and addressing medical issues before they develop into unmanageable problems. Programs such as IBM Watson are currently used for pattern recognition as part of diagnostics. Machine learning algorithms are more effective than human analysis in locating and diagnosing cancer. In fact, Watson can diagnose lung cancer in 90% of cases.
The truth behind the data
A common issue with medical research is that it typically depends on surveys, in which the patients interviewed report their own behaviors and habits. Consequently, many patients conceal the truth because they are concerned about their image. For instance, a patient tends to hesitate to declare that he does not properly follow a treatment. Obtaining good data with opinion therefore becomes difficult.
On the contrary, data that directly comes from a smartphone or a smartwatch cannot lie: such type of data is neutral and objective.
In health like in data, Treatment is the key
With Big Data, the main issue is not subjectivity but treatment. With so many captors, data collected from different processes and in various contexts, the volume of data in healthcare is indeed gigantic. The volume of Big Data in healthcare is expected to reach 2,312 exabytes in 2020.
The challenge therefore lies not in data but in humans. Data specialists (data scientists, data engineers, chief data officers) who can analyze and interpret massive volumes of data require extensive training.
In this era of deep digital transformation where experts with dual competencies are needed, emlyon business school’s MSc in Health Management & Data Intelligence provides graduate students with the strategic skills to navigate the challenges of the health sector.
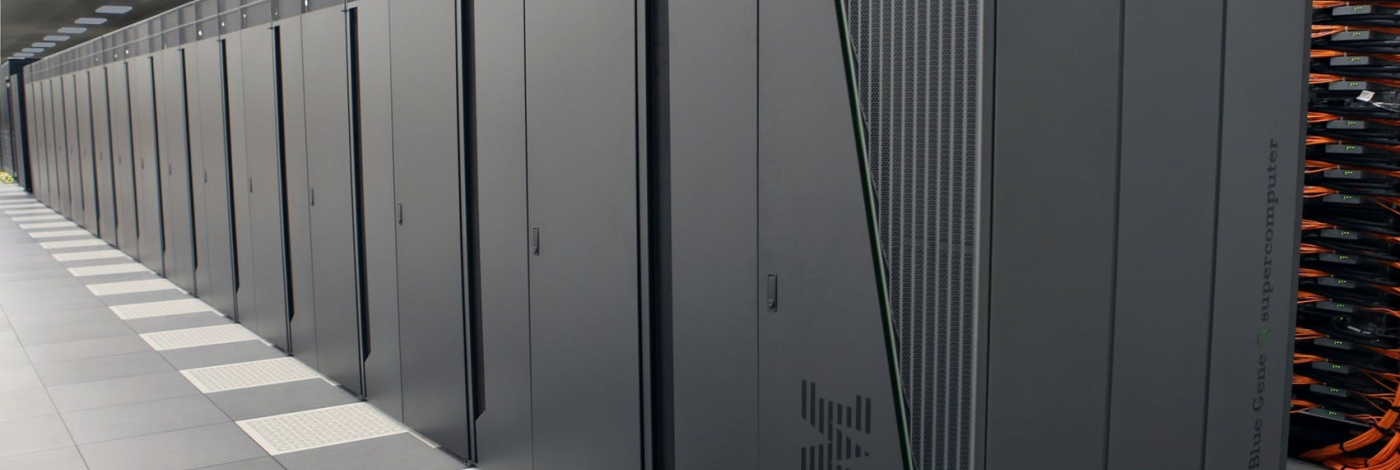
Oncologists at the Maine Center for Cancer Medicine and Westmed Medical Group in New York have started to test the IBM Watson supercomputer system in an effort to recommend treatment for lung cancer
Major hindrances in accessing the data
The development and usage of data in healthcare present a few obstacles.
First, the variety of data sources is a major issue for data scientists, as data is spread across many sources, including hospitals, states, administrative departments and now personal devices. Hence, the integration of these data sources is essential, which requires infrastructure where all data providers collaborate with each other.
Second, the sensitivity of healthcare data is important to consider. People are careful about their personal data, but some data are more critical than others. This case is especially true for bank and health data.
Grey areas
Despite the promising role of Big Data in healthcare, viewing it as the Holy Grail of healthcare is probably effusive.
A recent study of MS Househ and its colleagues entitled “Big Data, Big Problems: A Healthcare Perspective.” made waves for its provocative findings. Its conclusion is particularly quite scathing: “Our study results show that although Big Data is built up to be the ‘Holy Grail’ for healthcare, small data techniques using traditional statistical methods are, in many cases, more accurate and can lead to more improved healthcare outcomes than Big Data methods. In sum, Big Data for healthcare may cause more problems for the healthcare industry than solutions, and in short, when it comes to the use of data in healthcare, ‘size isn’t everything.’”
Combine small and Big Data
This perspective is highly insightful: with every new technology, we have to take a step back and contemplate both its opportunities and risks.
With regard to Big Data in healthcare, the best approach is probably to bear in mind that the solution to health issues can be found not only in Big Data but also in small data. Merging these two types of data therefore requires the most valuable human skill: the ability to reassess each situation according to the context.
Learn to use small and big data according to each context is the core of our new MSc in Health Management & Data Intelligence.